Wind power turbines are an essential component of renewable energy generation, converting the kinetic energy of wind into electrical energy. The performance and efficiency of wind turbines can be quantified using various advanced metrics and data points, providing valuable insights for optimizing their operation and maximizing energy output.
Wind Speed and Direction: The Driving Forces
Wind speed and direction are critical factors affecting the performance of wind turbines. As wind speed increases, the power generated by the wind turbine also increases, following a non-linear relationship. This relationship is typically described by the power curve, which can be used to estimate the turbine’s power output at different wind speeds.
The wind speed at the hub height of the turbine is a crucial parameter, as it directly impacts the kinetic energy available for conversion. Anemometers are commonly used to measure the wind speed, with high-precision instruments capable of recording data at a sampling rate of up to 50 Hz. This high-resolution data can be used to analyze the turbulence intensity, wind shear, and other important characteristics of the wind resource.
Wind direction is equally important, as wind turbines are designed to be oriented towards the prevailing wind direction to maximize energy capture. Wind vanes are used to measure the wind direction, and the data is used to adjust the turbine’s yaw angle to maintain optimal alignment with the wind.
Power Curve: Mapping the Efficiency Landscape
The power curve is a crucial metric that describes the relationship between wind speed and the power output of a wind turbine. It provides insights into the efficiency of the turbine across a range of wind speeds, allowing for the identification of the optimal operating conditions.
The power curve is typically obtained through field measurements or simulations, and it can be influenced by various factors, such as the turbine’s design, blade geometry, and environmental conditions. Advanced power curve models, such as those based on machine learning algorithms, can capture the non-linear and complex relationships between wind speed and power output, enabling more accurate performance predictions.
Performance Coefficient (Cp): Quantifying Efficiency
The performance coefficient (Cp) is a dimensionless parameter that quantifies the efficiency of a wind turbine. It is the ratio of the actual power output to the theoretical maximum power output that could be achieved by a wind turbine at a given wind speed.
The Cp value can range from 0 to the Betz limit of 0.593, which represents the maximum theoretical efficiency of a wind turbine. In practice, modern wind turbines can achieve Cp values in the range of 0.4 to 0.5, indicating that they are able to capture a significant portion of the available wind energy.
Factors such as blade design, rotor diameter, and tip speed ratio can influence the Cp value, and understanding these relationships is crucial for optimizing the turbine’s performance.
Rotor Speed: Balancing Power and Stability
The rotor speed of a wind turbine is another important metric that can impact its performance. The rotor speed is usually controlled by the turbine’s controller to maintain optimal power output and avoid damage to the turbine components.
At low wind speeds, the rotor speed is typically increased to maximize the power output, while at high wind speeds, the rotor speed is reduced to limit the power and prevent overloading the turbine. Advanced control algorithms, such as those based on model predictive control or fuzzy logic, can be used to optimize the rotor speed and maintain the turbine’s stability under varying wind conditions.
Air Density: The Invisible Influencer
Air density is a critical factor that affects the power output of a wind turbine. As air density increases, the mass flow rate of air through the turbine blades also increases, leading to higher power output. Conversely, lower air density, such as at higher altitudes, can result in reduced power generation.
Air density can be measured using barometers and temperature sensors, and the data can be used to adjust the turbine’s power curve and performance calculations. Accurate air density measurements are particularly important for offshore wind farms, where the air density can vary significantly due to changes in temperature and humidity.
Uncertainty Quantification: Embracing the Unknown
Uncertainty quantification is an essential aspect of wind turbine performance assessment. It involves estimating the uncertainty associated with the various parameters that affect wind turbine performance, such as wind speed, direction, and air density.
By quantifying the uncertainty, wind turbine operators can make more informed decisions about maintenance, operations, and investment strategies. Advanced statistical techniques, such as Monte Carlo simulations and Bayesian analysis, can be used to model the uncertainty and its impact on the turbine’s performance.
Incorporating uncertainty quantification into the performance assessment process can help wind turbine operators identify potential risks, optimize maintenance schedules, and improve the overall reliability and efficiency of their wind power systems.
Measurement Tools and Data Analytics
To measure the various parameters that affect wind turbine performance, a range of tools and techniques can be used, including:
- Anemometers: High-precision instruments for measuring wind speed
- Wind vanes: Devices for measuring wind direction
- Barometers: Instruments for measuring air pressure and density
- Meteorological sensors: Comprehensive systems for monitoring environmental conditions
- Data acquisition systems: Hardware and software for collecting and storing performance data
The data collected from these instruments can be analyzed using advanced statistical methods, machine learning algorithms, and other data analytics techniques. This can provide valuable insights into the turbine’s performance, identify areas for improvement, and support decision-making processes.
By leveraging these measurement tools and data analytics capabilities, wind turbine operators can optimize the performance and efficiency of their wind power systems, ultimately contributing to the growth and sustainability of the renewable energy sector.
References:
– National Renewable Energy Laboratory (NREL) – Wind Data and Tools
– National Science Foundation – Award Search: Wind Turbine Performance Quantification
– Iberdrola – Wind Measurement in Onshore Wind Energy
– Frontiers in Energy Research – Data-Driven Performance Quantification of a Vertical Axis Wind Turbine
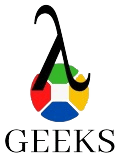
The lambdageeks.com Core SME Team is a group of experienced subject matter experts from diverse scientific and technical fields including Physics, Chemistry, Technology,Electronics & Electrical Engineering, Automotive, Mechanical Engineering. Our team collaborates to create high-quality, well-researched articles on a wide range of science and technology topics for the lambdageeks.com website.
All Our Senior SME are having more than 7 Years of experience in the respective fields . They are either Working Industry Professionals or assocaited With different Universities. Refer Our Authors Page to get to know About our Core SMEs.