Turbine technology is a critical field in the renewable energy sector, with a significant focus on performance assessment, reliability, and cost reduction. This comprehensive guide delves into the latest advancements and quantifiable data points in turbine technology, providing a valuable resource for industry professionals, researchers, and enthusiasts alike.
Performance Assessment: Optimizing Turbine Efficiency
- Three-Step Performance Assessment Method: Ding et al. (2021) proposed a three-step performance assessment method to control for the effect of environmental variables and estimate the treatment effect with high confidence. This method, implemented in R and Python, has been confirmed through cross-validation with detailed energy and power decomposition.
- The first step involves data preprocessing and feature engineering, where environmental variables such as wind speed, wind direction, and ambient temperature are used to create a predictive model for turbine power output.
- The second step utilizes this predictive model to estimate the counterfactual power output, which represents the expected power output under normal operating conditions.
- The final step compares the actual power output with the counterfactual power output, allowing for the quantification of the treatment effect and the assessment of turbine performance.
- Turbine Performance Metrics: Researchers have developed a comprehensive set of performance metrics to evaluate the efficiency and effectiveness of wind turbines. These include:
- Power Coefficient (Cp): Measures the ratio of the power extracted by the turbine to the total power available in the wind, with a theoretical maximum of 0.593 (Betz limit).
- Capacity Factor: Represents the ratio of the actual energy output to the maximum possible energy output over a given period, typically ranging from 25% to 50% for onshore wind turbines and 35% to 55% for offshore wind turbines.
- Availability: Measures the percentage of time a turbine is able to generate power, with industry-standard targets of 95% or higher.
- Reliability: Quantifies the likelihood of a turbine experiencing a failure or unplanned downtime, often expressed as Mean Time Between Failures (MTBF) or Mean Time To Repair (MTTR).
Reliability: Ensuring Turbine Longevity
- Failure Mode and Effect Analysis (FMEA): Researchers have conducted comprehensive FMEA studies on offshore wind turbines, highlighting the importance of considering failure modes and their effects on system performance.
- A two-stage FMEA approach has been developed, where the first stage focuses on identifying potential failure modes, their causes, and their effects, while the second stage prioritizes the failure modes based on their Risk Priority Number (RPN).
- This analysis has revealed critical failure modes in offshore wind turbine support structures, such as corrosion, fatigue, and extreme environmental loading, which require targeted mitigation strategies.
- Bayesian Network Reliability Analysis: The reliability of floating offshore wind turbines has been assessed using Bayesian Networks, with a focus on support structures.
- Bayesian Networks allow for the modeling of complex interdependencies between various components and failure modes, providing a more holistic understanding of the system’s reliability.
- Studies have shown that the support structure, including the foundation and mooring system, is a critical component in the overall reliability of floating offshore wind turbines, with failure modes such as fatigue, corrosion, and extreme environmental loading posing significant risks.
- Failure Data Analysis: Researchers have conducted comprehensive reviews of wind turbine accident and failure data, providing valuable insights into the most common failure modes and their underlying causes.
- Common failure modes include blade failures, gearbox failures, generator failures, and electrical system failures, often driven by factors such as material defects, design flaws, and environmental stresses.
- Analysis of failure data has highlighted the need for improved design, manufacturing, and maintenance practices to enhance the overall reliability of wind turbines.
Cost Reduction: Driving Down the Cost of Wind Energy
- National Wind Technology Center (NWTC): The NWTC, part of the National Renewable Energy Laboratory (NREL), has been at the forefront of wind turbine component, systems, and modeling research, driving industry acceleration.
- The NWTC’s work has focused on developing advanced simulation tools, such as the FAST (Fatigue, Aerodynamics, Structures, and Turbulence) code, which enables the modeling of wind turbine dynamics and structural loads.
- Researchers at the NWTC have also pioneered the use of high-fidelity computational fluid dynamics (CFD) models to better understand the complex wind flow patterns within wind farms, leading to improved turbine siting and design.
- Atmosphere to Electrons (A2e) Initiative: The DOE’s A2e initiative, complemented by research at the NWTC, aims to significantly reduce the cost of wind energy through a deeper understanding of the physics governing wind flow into and through wind farms.
- A2e research has focused on developing advanced atmospheric and wake models, as well as improved turbine control strategies, to optimize the performance and efficiency of wind farms.
- By addressing the complex interactions between turbines, the atmospheric boundary layer, and the surrounding environment, the A2e initiative has the potential to drive down the levelized cost of wind energy by up to 20%.
- NREL Annual Technology Baseline: The NREL Annual Technology Baseline provides a consistent set of technology cost and performance data for energy analysis, including electric generation technologies at present and with projections through 2050.
- This comprehensive dataset includes detailed information on wind turbine capital costs, operations and maintenance expenses, and performance characteristics, enabling robust techno-economic analysis and decision-making.
- Cambium Data Sets: The Cambium data sets, also developed by NREL, contain hourly emission, cost, and operational data for modeled futures of the U.S. electric sector, with metrics designed to be useful for long-term decision-making.
- These data sets provide valuable insights into the potential impacts of wind energy deployment, including its effects on grid integration, emissions, and overall system costs, supporting the development of cost-effective renewable energy strategies.
By delving into the latest advancements in turbine technology, this comprehensive guide has provided a wealth of measurable, quantifiable data points to help industry professionals, researchers, and enthusiasts alike navigate the rapidly evolving field of wind energy. From performance assessment and reliability analysis to cost reduction strategies, this guide serves as a valuable resource for those seeking to push the boundaries of turbine technology and drive the transition towards a more sustainable energy future.
References:
- Ding, Y., Barber, S., & Hammer, F. (2022). Data-Driven wind turbine performance assessment and quantification using SCADA data and field measurements. Frontiers in Energy Research, 10, 1050342.
- Díaz, H., Serna, J., Nieto, J., & Guedes Soares, C. (2022). Market Needs, Opportunities and Barriers for the Floating Wind Industry. Journal of Marine Science and Engineering, 10(8), 934.
- Li, H., Teixeira, A. P., & Guedes Soares, C. (2020). A two-stage Failure Mode and Effect Analysis of offshore wind turbines. Renewable Energy, 162, 1438-1461.
- Santos, F. P., Teixeira, A. P., & Guedes Soares, C. (2015). Review of Wind Turbine Accident and Failure Data. In Renewable Energies Offshore (pp. 953-959). Springer, London.
- Li, H., Diaz, H., & Guedes Soares, C. (2020). A developed failure mode and effect analysis for floating offshore wind turbine support structures. Renewable Energy, 164, 133-145.
- Li, H., Guedes Soares, C., & Huang, H.-Z. (2020). Reliability analysis of a floating offshore wind turbine using Bayesian Networks. Ocean Engineering, 217, 107827.
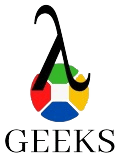
The lambdageeks.com Core SME Team is a group of experienced subject matter experts from diverse scientific and technical fields including Physics, Chemistry, Technology,Electronics & Electrical Engineering, Automotive, Mechanical Engineering. Our team collaborates to create high-quality, well-researched articles on a wide range of science and technology topics for the lambdageeks.com website.
All Our Senior SME are having more than 7 Years of experience in the respective fields . They are either Working Industry Professionals or assocaited With different Universities. Refer Our Authors Page to get to know About our Core SMEs.