The surprising dynamics of high-performing teams can be quantified and measured using various metrics and methods, providing valuable insights into the factors that contribute to a team’s success. This comprehensive guide delves into the technical details and specific applications of these metrics, equipping physics students with the knowledge to understand and optimize the dynamics of their own teams.
Measuring Communication Patterns: The Key to Team Success
According to the Harvard Business Review, call centers are an ideal starting point for studying the dynamics of high-performing teams due to the distinct, readily recruitable skills required for the job, the unambiguous and straightforward duties, and the quantifiable productivity metrics such as the number of problems resolved, customer satisfaction ratings, and average handling time (AHT).
In a study conducted by MIT’s Human Performance Dynamics Laboratory, communication patterns were found to be the most important predictor of a team’s success, contributing as much as all other factors combined. Successful teams share several defining characteristics in their communication style, including:
-
Equal Participation in Conversations: The degree of equality in the distribution of conversational turn-taking among team members is a key indicator of team performance. Teams with more equal participation tend to outperform those with unequal participation.
-
Energetic Conversations and Gestures: High-performing teams engage in more energetic and animated conversations, with team members using more hand gestures and body language to convey their ideas.
-
Direct Connections between Team Members: Successful teams exhibit a higher degree of direct communication between team members, with fewer intermediaries or “hubs” that channel the flow of information.
-
Back-channel or Side Conversations: Thriving teams often engage in back-channel or side conversations, which can facilitate the exchange of ideas and the development of shared understanding.
-
Exploration Outside the Team: High-performing teams are more likely to explore and connect with individuals and resources outside their immediate team, which can lead to the introduction of new ideas and perspectives.
To quantify these communication patterns, researchers have developed various metrics and techniques, such as:
- Conversational Turn-taking Entropy: This metric measures the degree of equality in the distribution of conversational turns among team members. Higher entropy indicates more equal participation.
- Gestural Expressiveness: This metric quantifies the energy and animation of team members’ gestures and body language during conversations.
- Network Centrality: This metric analyzes the structure of communication within the team, identifying the degree of direct connections between members and the presence of central “hubs.”
- Back-channel Ratio: This metric measures the proportion of side conversations or back-channel communication within the overall team communication.
- External Exploration: This metric tracks the frequency and extent of team members’ interactions with individuals and resources outside the immediate team.
By analyzing these communication patterns, researchers have found that individual reasoning and talent contribute far less to team success than one might expect. Instead, the best way to build a great team is to shape and guide the team’s communication patterns.
Actionable Insights: Driving Strategic Decision-making
In addition to the communication patterns, actionable insights can be derived from data to guide strategic decision-making. Actionable insights go beyond presenting data and provide context, implications, and recommendations for specific actions. They offer a clear direction on what to do next, based on a thorough understanding of the data at hand.
To derive actionable insights, researchers and practitioners can employ a range of techniques, such as:
-
Root Cause Analysis: This technique involves systematically identifying the underlying factors that contribute to a particular outcome or problem, allowing for targeted interventions.
-
Scenario Planning: By considering multiple possible future scenarios, teams can develop robust strategies that are adaptable to a range of circumstances.
-
Predictive Modeling: Advanced statistical and machine learning techniques can be used to build predictive models that forecast future team performance or outcomes based on current data.
-
Optimization Algorithms: Optimization algorithms can be used to identify the optimal configuration of team members, communication patterns, or other variables to maximize team performance.
-
Sensitivity Analysis: This technique involves systematically varying input parameters to understand their impact on team outcomes, enabling the identification of critical leverage points.
By leveraging these techniques, teams can derive actionable insights that inform strategic decision-making and guide the development of high-performing teams.
Dynamical O-information: Quantifying High-order Interdependencies
In the field of physics, the dynamical O-information framework is used to quantify high-order interdependencies via a multivariate extension of the mutual information. This framework allows for the separation of multiplets of variables that influence the future of the system synergistically from redundant multiplets.
The dynamical O-information is defined as:
O(X_1, X_2, ..., X_n) = I(X_1, X_2, ..., X_n; X_1', X_2', ..., X_n')
where X_1, X_2, ..., X_n
are the current state variables, and X_1', X_2', ..., X_n'
are the future state variables. The mutual information I(X_1, X_2, ..., X_n; X_1', X_2', ..., X_n')
quantifies the amount of information that the current state variables provide about the future state variables.
The dynamical O-information framework can be used to analyze the high-order interdependencies within a team, identifying the critical variables and their synergistic influence on the team’s future performance. This can provide valuable insights into the underlying dynamics that drive team success.
For example, consider a team of physicists working on a complex research project. The dynamical O-information framework could be used to analyze the interactions between team members, their communication patterns, the distribution of tasks, and the flow of information. By identifying the high-order interdependencies among these variables, the team can optimize their collaboration and decision-making processes to enhance their overall performance.
Practical Applications and Examples
To illustrate the practical applications of the concepts discussed in this guide, let’s consider a few examples:
- Optimizing Communication Patterns in a Physics Research Team:
- Analyze the communication patterns within a physics research team using the metrics discussed earlier, such as conversational turn-taking entropy, gestural expressiveness, and network centrality.
- Identify areas for improvement, such as unequal participation or the presence of communication “hubs.”
- Implement strategies to shape the team’s communication patterns, such as encouraging more equal participation, fostering energetic discussions, and promoting direct connections between team members.
-
Measure the impact of these interventions on the team’s productivity, problem-solving abilities, and overall performance.
-
Deriving Actionable Insights from Team Performance Data:
- Collect data on the team’s performance metrics, such as the number of research papers published, the success rate of grant applications, and the timeliness of project deliverables.
- Employ techniques like root cause analysis, scenario planning, and predictive modeling to identify the key factors driving team performance.
- Develop actionable insights that provide clear recommendations for improving team processes, resource allocation, and strategic decision-making.
-
Implement the recommended actions and monitor the team’s progress over time.
-
Analyzing High-order Interdependencies in a Multidisciplinary Physics Team:
- Assemble a multidisciplinary team of physicists, each with their own area of expertise (e.g., theoretical physics, experimental physics, computational physics).
- Apply the dynamical O-information framework to quantify the high-order interdependencies among the team members, their communication patterns, the distribution of tasks, and the flow of information.
- Identify the critical variables and their synergistic influence on the team’s overall performance.
- Optimize the team’s structure, communication channels, and decision-making processes to leverage the identified high-order interdependencies and enhance the team’s productivity and innovation.
By applying the concepts and techniques discussed in this guide, physics students can develop a deeper understanding of the surprising dynamics that underlie high-performing teams and leverage this knowledge to build and improve their own teams for greater success.
References:
- Harvard Business Review: The New Science of Building Great Teams
- Yellow Training: What Kind of Communication Makes a Team Great?
- Sopact: Actionable Insights: What They Are and How to Use Them
- NCBI: Dynamical O-information: Quantifying Synergistic Interdependencies
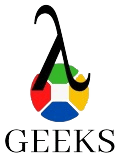
The lambdageeks.com Core SME Team is a group of experienced subject matter experts from diverse scientific and technical fields including Physics, Chemistry, Technology,Electronics & Electrical Engineering, Automotive, Mechanical Engineering. Our team collaborates to create high-quality, well-researched articles on a wide range of science and technology topics for the lambdageeks.com website.
All Our Senior SME are having more than 7 Years of experience in the respective fields . They are either Working Industry Professionals or assocaited With different Universities. Refer Our Authors Page to get to know About our Core SMEs.