Stacking in microscopy is a powerful technique that combines multiple images taken at different focal planes to create a single, in-focus image. This process is particularly useful for capturing detailed information from samples with varying depths, such as biological specimens or complex structures. In this comprehensive guide, we will delve into the intricacies of stacking in microscopy, providing you with a wealth of technical details, formulas, and practical examples to help you master this essential skill.
Understanding the FocusALL Technique
The FocusALL technique is a widely used method for determining the in-focus regions of an image. This approach employs the Modified Harris Corner Response Measure, which is a robust algorithm that can effectively identify the sharpest areas within an image, even in the presence of high contrast or high-intensity regions that may not be in perfect focus.
The Modified Harris Corner Response Measure is defined as:
R = det(M) - k * trace(M)^2
where M
is the 2×2 structure tensor, and k
is a constant typically set between 0.04 and 0.06. The regions with the highest R
values are considered to be the most in-focus areas of the image.
By utilizing the FocusALL technique, you can ensure that your stacked images accurately represent the true focus of your sample, even in challenging scenarios.
Optimizing Image Acquisition
Proper image acquisition is crucial for successful stacking in microscopy. Here are some key considerations:
-
Lossless File Formats: When capturing images, it is essential to use lossless file formats such as PNG, TIFF, or GIF. These formats preserve the image quality without compromising data during compression, which is vital for automated image analysis.
-
Exposure Time: Ensuring the proper exposure time is critical to avoid saturation and maintain a good dynamic range. Overexposure can lead to loss of detail, while underexposure can result in poor signal-to-noise ratios.
-
Binning: Binning is a technique that combines the signal from nearby pixels, effectively increasing the signal-to-noise ratio. However, this process can also lead to a loss of information about small objects within the sample. It is essential to find the right balance between signal-to-noise improvement and preserving spatial resolution.
To illustrate the impact of binning, consider the following example:
Pixel size without binning: 0.1 μm
Pixel size with 2x2 binning: 0.2 μm
In this case, the binning process has doubled the pixel size, potentially obscuring the details of smaller structures within the sample.
Quantifying Confocal Fluorescent Images
Quantifying confocal fluorescent images is a crucial step in many microscopy-based studies. Two key metrics to consider are:
-
Mean Fluorescent Intensity: This measure is used to quantify the relative levels of a molecule of interest across a region of interest. It is calculated as the average intensity of all pixels within the selected region.
-
Cell Number and Percentage of Positive Cells: These metrics are used to quantify the number of cells and the percentage of cells that are positive for a specific stain. This information is essential for understanding the distribution and abundance of target molecules or cell populations within the sample.
To illustrate the calculation of these metrics, let’s consider a hypothetical example:
Suppose we have a confocal fluorescent image of a cell culture, and we want to quantify the expression of a specific protein. We can use the following steps:
- Define the regions of interest (ROIs) corresponding to individual cells.
- Calculate the mean fluorescent intensity for each ROI using the formula:
Mean Fluorescent Intensity = Σ Pixel Intensities / Number of Pixels in ROI
- Determine the number of cells that are positive for the protein of interest based on a predetermined intensity threshold.
- Calculate the percentage of positive cells as:
Percentage of Positive Cells = (Number of Positive Cells / Total Number of Cells) × 100
These quantitative metrics provide valuable insights into the distribution and expression levels of target molecules within the sample, which is crucial for understanding biological processes and evaluating the effects of experimental treatments.
Extracting Meaningful Data from Microscopy Images
Microscopy data can be a rich source of information, but it is essential to understand the different types of data that can be extracted and the factors that affect their relevance in downstream analysis. The three main types of information that can be obtained from microscopy data are:
-
Intensity: This refers to the brightness or luminance of the pixels in the image, which can be used to quantify the levels of a specific molecule or structure.
-
Morphology: This encompasses the size, shape, and spatial arrangement of objects within the image, such as cells, organelles, or other biological structures.
-
Object Counts: This involves the identification and enumeration of individual objects, such as cells or particles, within the image.
Each of these data types has its own methods of measurement and factors that can influence their relevance in subsequent analyses. For example, factors like illumination, sample preparation, and image processing algorithms can all impact the accuracy and reliability of the extracted data.
To ensure the validity and reproducibility of your microscopy data, it is crucial to carefully consider these factors and implement appropriate quality control measures throughout the entire experimental workflow.
Enhancing Image Processing Algorithms
Stacking in microscopy relies on advanced image processing algorithms to combine multiple images into a single, in-focus result. These algorithms can be further enhanced to handle high-resolution images and varying illumination conditions.
One such algorithm is the Focal Stacking Algorithm, which uses a weighted average of the pixel intensities from the input images to create the final stacked image. The weighting factor is typically determined by the sharpness or focus measure of each pixel, as calculated using techniques like the Modified Harris Corner Response Measure.
Another important image processing technique is Image Denoising, which aims to remove unwanted noise and artifacts from the microscopy images. One effective method for this is the BaSiC (Background and Shading Correction) algorithm, which can correct for uneven illumination and background variations in optical microscopy images.
By leveraging these advanced image processing algorithms, you can enhance the quality and accuracy of your stacked microscopy images, enabling more reliable and informative data analysis.
Automated Image Analysis Tools
To streamline the analysis of microscopy data, several software tools have been developed that offer powerful automated image analysis capabilities. Two prominent examples are:
-
CellProfiler: This open-source software platform is designed for automated image analysis, including tasks such as spot detection, object segmentation, and image correction. CellProfiler provides a user-friendly interface and a wide range of built-in modules to facilitate the analysis of complex microscopy data.
-
FIJI-ImageJ: FIJI (Fiji Is Just ImageJ) is an enhanced distribution of ImageJ, a popular open-source platform for biological image analysis. FIJI-ImageJ offers a comprehensive suite of tools for quantifying fluorescent images, measuring morphological features, and performing advanced image processing tasks.
These software tools can greatly simplify and accelerate the analysis of your stacked microscopy images, allowing you to extract valuable insights from your data more efficiently.
Conclusion
Stacking in microscopy is a powerful technique that can significantly improve the quality and clarity of your microscopy images. By understanding the underlying principles, optimizing your image acquisition, and leveraging advanced image processing algorithms and analysis tools, you can unlock the full potential of this essential skill.
Remember, the key to success in stacking microscopy lies in attention to detail, a deep understanding of the underlying physics and mathematics, and a willingness to experiment and refine your approach. With the guidance provided in this comprehensive guide, you are well on your way to mastering the art of stacking in microscopy.
References:
- Sigdel, M. S., Sigdel, M., Dinç, S., Dinç, I., Pusey, M. L., & Aygün, R. S. (2016). FocusALL: Focal Stacking of Microscopic Images Using Modified Harris Corner Response Measure. Journal of Microscopy and Ultrastructure, 4(2), 1–9. doi: 10.1016/j.jmau.2016.04.001
- Carpenter, A. (2017). Quantifying microscopy images: top 10 tips for image acquisition. Carpenter-Singh Lab Blog. Retrieved from https://carpenter-singh-lab.broadinstitute.org/blog/quantifying-microscopy-images-top-10-tips-for-image-acquisition
- Mahbubul, S., Novo, S. G., Le Marchand, S. J., Wang, Y., & Duncan, M. K. (2021). A simple method for quantitating confocal fluorescent images. Matrix Biology, 96, 102–113. doi: 10.1016/j.matbio.2020.02.004
- Culley, S., Caballero, A., Cuber, B., Uhlmann, V., & Burden, J. J. (2023). Made to measure: An introduction to quantifying microscopy data in the life sciences. Journal of Microscopy and Imaging, 132(8), 1–12. doi: 10.1111/jmi.13208
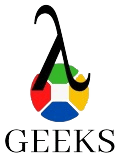
The lambdageeks.com Core SME Team is a group of experienced subject matter experts from diverse scientific and technical fields including Physics, Chemistry, Technology,Electronics & Electrical Engineering, Automotive, Mechanical Engineering. Our team collaborates to create high-quality, well-researched articles on a wide range of science and technology topics for the lambdageeks.com website.
All Our Senior SME are having more than 7 Years of experience in the respective fields . They are either Working Industry Professionals or assocaited With different Universities. Refer Our Authors Page to get to know About our Core SMEs.