Lithium density 2, also known as lithium-ion battery density, refers to the amount of energy that can be stored in a given volume or weight of a lithium-ion battery. This parameter is crucial in the design and optimization of lithium-ion batteries for various applications, such as electric vehicles, portable electronics, and renewable energy storage systems.
Measuring Lithium Ion Battery Density
Inductively Coupled Plasma Optical Emission Spectroscopy (ICP-OES)
One common method for measuring lithium-ion battery density is Inductively Coupled Plasma Optical Emission Spectroscopy (ICP-OES). This technique can analyze the elemental composition of lithium-ion battery materials, including the concentration of lithium and other elements, such as:
- Lithium manganese oxide (LiMn2O4)
- Lithium carbonate (Li2CO3)
- Lithium chloride (LiCl)
- Lithium dihydrogen phosphate (LiH2PO4)
- Lithium oxide (Li2O)
The data obtained from ICP-OES can be used to calculate the lithium-ion battery density.
ICP-OES Principle and Procedure
The ICP-OES technique works by ionizing the sample material in a high-temperature plasma and then analyzing the emitted light spectrum to determine the elemental composition. The general procedure for using ICP-OES to measure lithium-ion battery density is as follows:
- Sample Preparation: The lithium-ion battery materials are dissolved in an appropriate solvent, such as nitric acid or hydrochloric acid, to create a homogeneous solution.
- Instrument Calibration: The ICP-OES instrument is calibrated using standard solutions with known concentrations of the elements of interest, such as lithium.
- Sample Analysis: The prepared sample solution is introduced into the ICP-OES instrument, where it is atomized and ionized in the high-temperature plasma. The emitted light spectrum is then analyzed to determine the concentration of lithium and other elements.
- Data Analysis: The measured concentrations of lithium and other elements are used to calculate the lithium-ion battery density, taking into account the specific chemical composition and stoichiometry of the battery materials.
Electrochemical Impedance Spectroscopy (EIS) and Incremental Capacity-Differential Voltage (IC-DV)
Another method for measuring lithium-ion battery density is using Electrochemical Impedance Spectroscopy (EIS) and Incremental Capacity-Differential Voltage (IC-DV) techniques. These techniques can provide information about the electrochemical behavior and kinetics of lithium-ion batteries, which can be used to estimate the battery density.
EIS Principle and Procedure
EIS is a non-destructive technique that measures the impedance of a lithium-ion battery over a range of frequencies. The impedance data can be used to analyze the charge transfer resistance, diffusion resistance, and other electrochemical properties of the battery, which are related to the battery density.
The general procedure for using EIS to measure lithium-ion battery density is as follows:
- Battery Preparation: The lithium-ion battery is prepared for EIS measurement, which may involve charging or discharging the battery to a specific state of charge.
- EIS Measurement: The battery is connected to an EIS instrument, and a small AC signal is applied to the battery over a range of frequencies, typically from 0.1 Hz to 100 kHz.
- Data Analysis: The measured impedance data is analyzed using equivalent circuit models or other data analysis techniques to extract parameters related to the battery density, such as charge transfer resistance and diffusion resistance.
IC-DV Principle and Procedure
IC-DV is a technique that measures the incremental capacity (dQ/dV) and differential voltage (dV/dQ) of a lithium-ion battery during charge and discharge cycles. These parameters can provide information about the electrochemical processes occurring within the battery, which can be used to estimate the battery density.
The general procedure for using IC-DV to measure lithium-ion battery density is as follows:
- Battery Cycling: The lithium-ion battery is cycled through charge and discharge cycles, typically at a constant current or constant power.
- Data Acquisition: The voltage and capacity of the battery are measured during the cycling process, and the incremental capacity (dQ/dV) and differential voltage (dV/dQ) are calculated.
- Data Analysis: The IC-DV data is analyzed to identify features, such as peaks and inflection points, which can be related to the electrochemical processes occurring within the battery and used to estimate the battery density.
Machine Learning and Data-Driven Approaches
In addition to the traditional measurement techniques, machine learning and data-driven approaches can also be used to predict and optimize lithium-ion battery density. These approaches can analyze large datasets of battery performance and characteristics, such as voltage, current, temperature, and capacity, to identify patterns and trends that can be used to improve battery design and performance.
Machine Learning for Lithium-Ion Battery Density Optimization
One example of a machine learning approach for lithium-ion battery density optimization is the study by Yadav et al., which used machine learning to predict the mass load of lithium-ion battery electrode materials based on process parameters, such as slurry composition, coating thickness, and calendaring pressure. The study showed that the predicted mass load was highly correlated with the experimental mass load, indicating the potential of machine learning for optimizing lithium-ion battery density.
Other machine learning techniques that can be applied to lithium-ion battery density optimization include:
- Artificial Neural Networks (ANNs)
- Support Vector Machines (SVMs)
- Random Forests
- Gradient Boosting Machines
These techniques can be used to develop predictive models that can estimate lithium-ion battery density based on various input parameters, such as material composition, manufacturing processes, and operating conditions.
Factors Affecting Lithium-Ion Battery Density
The lithium-ion battery density is influenced by several factors, including:
- Material Composition: The type and concentration of active materials, such as lithium, cobalt, nickel, and manganese, can significantly affect the battery density.
- Electrode Design: The thickness, porosity, and active material loading of the electrodes can impact the battery density.
- Cell Design: The design of the battery cell, including the size, shape, and packaging, can influence the overall battery density.
- Manufacturing Processes: The manufacturing processes, such as slurry preparation, coating, and calendaring, can affect the density of the electrode materials and the overall battery density.
- Operating Conditions: The temperature, state of charge, and cycling conditions can also impact the battery density, particularly during long-term use and aging.
Practical Applications and Considerations
Lithium-ion battery density is a critical parameter in the design and optimization of various applications, including:
- Electric Vehicles: Improving the lithium-ion battery density can increase the driving range and energy efficiency of electric vehicles.
- Portable Electronics: Higher lithium-ion battery density can enable smaller and lighter portable devices with longer battery life.
- Renewable Energy Storage: Lithium-ion batteries with high energy density are essential for efficient energy storage in renewable energy systems, such as solar and wind power.
When designing and optimizing lithium-ion batteries for these applications, it is important to consider factors such as safety, cost, and environmental impact, in addition to the battery density.
Conclusion
Lithium density 2, or lithium-ion battery density, is a crucial parameter in the design and optimization of lithium-ion batteries for various applications. This comprehensive guide has provided an in-depth overview of the methods and techniques used to measure and quantify lithium-ion battery density, including ICP-OES, EIS, IC-DV, and machine learning approaches. Additionally, the guide has discussed the factors that affect lithium-ion battery density and the practical applications and considerations for this important parameter.
By understanding the principles and techniques behind lithium-ion battery density measurement and optimization, physics students can gain valuable insights into the design and development of advanced energy storage systems, which are essential for the transition to a more sustainable and efficient energy future.
References
- A Practical Guide To Elemental Analysis of Lithium Ion Battery Materials Using ICP-OES. Agilent Technologies, Inc., 2023.
- Lithium-ion battery data and where to find it. ScienceDirect, 2021.
- Simulation and Measurement of the Current Density Distribution in Lithium-Ion Batteries by a Multi-Tab Cell Approach. IOPscience, 2017.
- Protocol for quantifying inactive lithium in anode-free lithium-metal batteries. Nature, 2022.
- A Comparison between Electrochemical Impedance Spectroscopy and Incremental Capacity-Differential Voltage as Li-ion Battery Diagnostic Tools. Journal of The Electrochemical Society, 2018.
- Mass load prediction for lithium-ion battery electrode clean production: A machine learning approach. Journal of Cleaner Production, 2020.
- Data-driven prediction of battery cycle life before capacity degradation. Nature Communications, 2019.
- Identification and machine learning prediction of knee-point and knee-onset in capacity degradation curves of lithium-ion batteries. Journal of Power Sources, 2020.
- Degradation of Commercial Lithium-Ion Cells as a Function of Chemistry and Cycling Conditions. Journal of The Electrochemical Society, 2019.
- Accelerated cycle life testing and capacity degradation modeling of LiCoO2-graphite cells. Journal of Power Sources, 2017.
- Cycle life testing and modeling of graphite/LiCoO2 cells under different state of charge ranges. Journal of Power Sources, 2016.
- A novel method for the modeling of the state of health of lithium-ion cells using machine learning for practical applications. Journal of Power Sources, 2015.
- Calendar Aging of Lithium-Ion Batteries: I. Capacity Fading. Journal of The Electrochemical Society, 2014.
- Lithium-ion capacitor – Characterization and development of new electrical model. Journal of Energy Storage, 2015.
- Identifying degradation patterns of lithium ion batteries from impedance spectroscopy using machine learning. Journal of Power Sources, 2019.
- Long-Term Low-Rate Cycling of LiCoO2/Graphite Li-Ion Cells at 55°C. Journal of The Electrochemical Society, 2016.
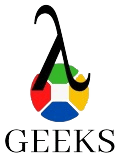
The lambdageeks.com Core SME Team is a group of experienced subject matter experts from diverse scientific and technical fields including Physics, Chemistry, Technology,Electronics & Electrical Engineering, Automotive, Mechanical Engineering. Our team collaborates to create high-quality, well-researched articles on a wide range of science and technology topics for the lambdageeks.com website.
All Our Senior SME are having more than 7 Years of experience in the respective fields . They are either Working Industry Professionals or assocaited With different Universities. Refer Our Authors Page to get to know About our Core SMEs.