Optimizing magnetic energy in magnetic bearings for high-speed machinery is a critical task that involves a deep understanding of electromagnetism, material properties, and optimization techniques. This comprehensive guide will provide you with the necessary knowledge and tools to effectively optimize the magnetic energy in your magnetic bearings, ensuring their reliable and efficient performance in high-speed machinery applications.
Material Selection for Magnetic Bearings
The selection of appropriate materials for the components of a magnetic bearing is crucial for optimizing its magnetic energy. The key material properties to consider are:
-
Magnetic Permeability: The magnetic permeability of the rotor and stator materials determines the ease with which the magnetic flux can pass through them. Materials with high magnetic permeability, such as soft magnetic alloys, are preferred to minimize magnetic reluctance and maximize the magnetic field strength.
-
Electrical Conductivity: The electrical conductivity of the rotor and stator materials affects the eddy current losses. Materials with low electrical conductivity, such as laminated steel or silicon-iron alloys, are preferred to reduce these losses and improve the bearing’s efficiency.
-
Saturation Flux Density: The saturation flux density of the permanent magnet material determines the maximum magnetic field strength that can be achieved. Materials with high saturation flux density, such as neodymium-iron-boron (NdFeB) magnets, are preferred to maximize the bearing capacity.
To illustrate the impact of material selection, let’s consider a numerical example. Suppose we have a 3-DOF hybrid magnetic bearing with a rotor made of a solid steel alloy and a stator made of a laminated silicon-iron alloy. The permanent magnets are made of NdFeB. The key material properties are:
- Rotor material: Magnetic permeability (μr) = 5000, Electrical conductivity (σ) = 2.2 × 10^6 S/m
- Stator material: Magnetic permeability (μr) = 3000, Electrical conductivity (σ) = 1.8 × 10^6 S/m
- Permanent magnet material: Saturation flux density (Bs) = 1.4 T
By selecting these materials, we can optimize the magnetic energy in the bearing by reducing eddy current losses and maximizing the magnetic field strength.
Geometric Design Optimization
The geometric design of the magnetic bearing components can significantly impact the magnetic energy optimization. Key design parameters to consider include:
-
Air Gap Minimization: Reducing the air gap between the rotor and stator can increase the magnetic flux density and improve the bearing capacity. This can be achieved by optimizing the shape and size of the rotor and stator.
-
Permanent Magnet Arrangement: The arrangement and number of permanent magnets can be optimized to maximize the magnetic field strength and bearing capacity. This may involve techniques such as Halbach array design.
-
Rotor and Stator Geometry: The shape and dimensions of the rotor and stator can be optimized to minimize eddy current losses and improve the overall bearing performance.
To illustrate the impact of geometric design optimization, let’s consider a numerical example. Suppose we have a 3-DOF hybrid magnetic bearing with the following initial design parameters:
- Air gap: 0.5 mm
- Permanent magnet arrangement: Radial magnetization
- Rotor diameter: 50 mm
- Stator inner diameter: 52 mm
By optimizing these design parameters, we can potentially reduce the air gap to 0.3 mm, arrange the permanent magnets in a Halbach array, and reduce the rotor diameter to 48 mm and the stator inner diameter to 50 mm. These changes can lead to a significant improvement in the bearing’s magnetic energy and performance.
Optimization Techniques
In addition to material selection and geometric design, optimization techniques can be employed to further enhance the magnetic energy in magnetic bearings. Some of the commonly used optimization techniques include:
-
Multi-Objective Optimization: Techniques like Non-dominated Sorting Genetic Algorithm (NSGA-II) can be used to optimize the bearing performance with respect to multiple objectives, such as maximum bearing capacity and minimum cost.
-
Gradient-Based Optimization: Methods like the adjoint method can be used to efficiently compute the gradients of the objective function with respect to the design variables, enabling rapid optimization of the bearing design.
-
Evolutionary Algorithms: Algorithms like Differential Evolution (DE) can be used to optimize the bearing design by exploring the design space and identifying the optimal configuration.
-
Surrogate Modeling: Techniques like Kriging or Radial Basis Function (RBF) can be used to build surrogate models of the bearing performance, which can then be used to efficiently explore the design space and identify the optimal configuration.
By applying these optimization techniques, you can further refine the material selection and geometric design of your magnetic bearing to achieve the desired level of magnetic energy optimization.
Dynamical Model Optimization
The dynamical model of the magnetic bearing can also be optimized to improve its performance and reduce the impact of eddy current effects. One approach is to use the axial Green function method for axisymmetric electromagnetic field computation, which can accurately model the electromagnetic field distribution and the resulting forces and torques acting on the bearing.
By optimizing the dynamical model, you can better understand the complex interactions between the rotor, stator, and permanent magnets, and make informed decisions to minimize eddy current losses and improve the overall bearing performance.
Conclusion
Optimizing magnetic energy in magnetic bearings for high-speed machinery is a multifaceted challenge that requires a deep understanding of electromagnetism, material properties, and optimization techniques. By carefully selecting the appropriate materials, optimizing the geometric design, applying advanced optimization techniques, and refining the dynamical model, you can achieve significant improvements in the magnetic energy and overall performance of your magnetic bearings.
This comprehensive guide has provided you with the necessary knowledge and tools to tackle this challenge effectively. Remember to continuously explore new advancements in the field and adapt your approach accordingly to ensure the reliable and efficient operation of your high-speed machinery.
References
- Evaluation Criteria of Material Selection on 3-DOF Hybrid Magnetic Bearing. https://doi.org/10.1109/tia.2021.3091657
- Design and Optimization Method of Magnetic Bearing for High-Speed Motor Considering Eddy Current Effects. https://doi.org/10.1109/tie.2017.2716875
- Multi-Objective Design Optimization of an IPMSM Based on Multilevel Strategy. https://doi.org/10.1109/tie.2020.2965463
- Multiobjective Optimization Design of Permanent Magnet Assisted Bearingless Synchronous Reluctance Motor Using NSGA-Ⅱ. https://ieeexplore.ieee.org/document/9604094
- Optimization of Radial Active Magnetic Bearings by Differential Evolution. https://link.springer.com/chapter/10.1007/3-540-31306-0_15
- Magnetic Bearing Design and Analysis for Structure Optimization in High-Speed Machinery. https://pubs.aip.org/aip/acp/article-pdf/doi/10.1063/5.0146889/18013823/140004_1_5.0146889.pdf
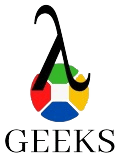
The lambdageeks.com Core SME Team is a group of experienced subject matter experts from diverse scientific and technical fields including Physics, Chemistry, Technology,Electronics & Electrical Engineering, Automotive, Mechanical Engineering. Our team collaborates to create high-quality, well-researched articles on a wide range of science and technology topics for the lambdageeks.com website.
All Our Senior SME are having more than 7 Years of experience in the respective fields . They are either Working Industry Professionals or assocaited With different Universities. Refer Our Authors Page to get to know About our Core SMEs.