Remote sensing is a powerful tool for quantifying the energy characteristics of the Earth’s surface, enabling researchers, policymakers, and energy professionals to make informed decisions. This comprehensive guide delves into the various methods and techniques used to measure energy in remote sensing applications, providing a detailed and technical overview for physics students and enthusiasts.
Radiometric Measurements: Quantifying Electromagnetic Radiation
Radiometric measurements are the foundation of energy assessment in remote sensing. These measurements involve the quantification of electromagnetic radiation in terms of its intensity, wavelength, and direction. The primary instruments used for this purpose are multispectral and hyperspectral imagers, which measure the reflected or emitted radiation in multiple spectral bands.
Spectral Radiance and Irradiance
The key radiometric quantities in remote sensing are spectral radiance and spectral irradiance. Spectral radiance (L) is the radiant flux per unit solid angle per unit projected area, measured in watts per square meter per steradian (W/m²/sr). Spectral irradiance (E) is the radiant flux per unit area, measured in watts per square meter (W/m²).
The relationship between spectral radiance and spectral irradiance is given by the equation:
E = π × L
where π is the constant approximately equal to 3.14159.
Sensor Calibration and Atmospheric Correction
To obtain accurate radiometric measurements, remote sensing instruments must be properly calibrated, and the effects of the atmosphere on the measured radiation must be accounted for. Sensor calibration involves the use of standard light sources and reference targets to establish the relationship between the sensor’s digital numbers and the corresponding radiometric quantities.
Atmospheric correction, on the other hand, involves the use of radiative transfer models to estimate the absorption and scattering of radiation by atmospheric constituents, such as gases, aerosols, and clouds. This correction is essential for retrieving the true surface reflectance or emittance from the measured at-sensor radiance.
Solar Radiation Estimation
The amount of solar radiation reaching the Earth’s surface is a critical factor in remote sensing applications, particularly for renewable energy systems. Several methods have been developed to estimate solar radiation using satellite-derived data and machine learning techniques.
Satellite-Derived Solar Radiation Predictors
Satellite-derived predictors, such as cloud cover, aerosol optical depth, and water vapor content, can be used to estimate solar radiation at the surface. These predictors are typically obtained from sensors like the Moderate Resolution Imaging Spectroradiometer (MODIS) and the Geostationary Operational Environmental Satellite (GOES) series.
Artificial Neural Networks for Solar Radiation Forecasting
Artificial neural networks (ANNs) have been widely used for solar radiation forecasting. These machine learning models can be trained on satellite data, ground-based measurements, and other meteorological variables to predict solar radiation with high accuracy, even in the absence of local telemetry data.
Numerical Weather Prediction Models
Numerical weather prediction (NWP) models, such as the Weather Research and Forecasting (WRF) model, can also be used to estimate solar radiation. These models incorporate satellite data, ground-based observations, and complex atmospheric physics to provide detailed solar radiation estimates at various spatial and temporal scales.
Building Energy Consumption Analysis
Remote sensing can be used to estimate the energy consumption of buildings by analyzing their thermal characteristics, materials, and surrounding environment. This information is crucial for optimizing energy efficiency and developing strategies to reduce energy consumption in the building sector.
Thermal Imaging and Building Envelope Analysis
Thermal infrared (IR) imaging can be used to detect heat signatures of buildings, providing insights into their thermal performance and energy efficiency. By analyzing the thermal characteristics of building envelopes, researchers can identify areas of heat loss or gain, which can inform retrofit strategies and energy-saving measures.
Multispectral and Hyperspectral Analysis of Building Materials
Multispectral and hyperspectral remote sensing can be used to identify the materials used in building construction, such as roofing, siding, and insulation. This information can be used to estimate the thermal properties of buildings and their energy performance.
Integration with GIS and Building Energy Modeling
Remote sensing data can be integrated with geographic information systems (GIS) and building energy modeling software to create comprehensive energy assessments. This approach allows for the analysis of building energy consumption at the neighborhood or city scale, enabling the development of energy-efficient urban planning strategies.
Vegetation and Land Cover Analysis
Remote sensing provides valuable information about vegetation and land cover, which are essential for understanding the energy balance of ecosystems and assessing the potential for renewable energy sources, such as biomass and wind energy.
Vegetation Indices and Biomass Estimation
Vegetation indices, such as the Normalized Difference Vegetation Index (NDVI) and the Enhanced Vegetation Index (EVI), can be used to estimate the health and productivity of vegetation. These indices, combined with other remote sensing data, can be used to assess the potential for biomass energy production.
Land Cover Classification and Renewable Energy Potential
Land cover classification, using techniques like supervised and unsupervised classification, can identify areas suitable for renewable energy development, such as wind farms or solar power plants. This information can be used to optimize the placement and design of these energy systems.
Integration with GIS for Renewable Energy Mapping
Remote sensing data can be integrated with GIS to create comprehensive maps of renewable energy resources, including solar irradiance, wind speed, and biomass availability. These maps can inform decision-making processes and guide the development of renewable energy projects.
Data Fusion and Machine Learning Techniques
Advanced data analysis techniques, such as data fusion and machine learning, can be used to extract energy-related information from large and complex remotely sensed datasets. These techniques can automate the extraction process, improve the accuracy and reliability of the results, and facilitate large-scale acquisition of critical energy system variables.
Data Fusion for Multimodal Analysis
Data fusion involves the integration of multiple remote sensing datasets, such as satellite imagery, LiDAR, and ground-based measurements, to provide a more comprehensive understanding of energy-related phenomena. This approach can enhance the accuracy and reliability of energy assessments.
Machine Learning for Automated Feature Extraction
Machine learning algorithms, including supervised and unsupervised methods, can be used to automate the extraction of energy-related features from remote sensing data. This includes the detection of solar photovoltaic panels, the estimation of building energy consumption, and the mapping of renewable energy resources.
Deep Learning for Solar Radiation Forecasting
Deep learning neural networks, trained on satellite-derived predictors, have shown promising results in long-term solar radiation forecasting. These models can provide accurate estimates of solar irradiance without the need for local telemetry data, enabling better planning and optimization of solar energy systems.
By understanding and applying these advanced techniques, physics students and enthusiasts can leverage the power of remote sensing to measure and analyze energy-related characteristics of the Earth’s surface, contributing to the development of sustainable energy solutions.
References
- Ren, S., Bradbury, W., Harrison-Atlas, K., Malaguzzi, D., Laura Murray, V., Malof, B., & Jordan M., (2022). Automated Extraction of Energy Systems Information from Remotely Sensed Data: A Review and Analysis. Remote Sensing, 14(22), 5790.
- Ren, S., Bradbury, W., Harrison-Atlas, K., Malaguzzi, D., Laura Murray, V., Malof, B., & Jordan M., (2022). Overview of Remotely Sensed Data Sources for Energy Applications. arXiv preprint arXiv:2202.12939.
- Remote Sensing – Week 11 Flashcards. Quizlet.
- Application of Satellite Sensor Data and Models for Energy Management. ResearchGate.
- The Laboratory for Applications of Remote Sensing. NASA.
- Estimation of shortwave solar radiation using the artificial neural network from Himawari-8 satellite imagery over China. Remote Sensing of Environment, 237, 111490.
- Deep Learning Neural Networks Trained with MODIS Satellite-Derived Predictors for Long-Term Global Solar Radiation. Remote Sensing, 11(21), 2564.
- Machine learning methods for solar radiation forecasting: A review. Renewable and Sustainable Energy Reviews, 65, 787-804.
- Short-term forecasting of solar irradiance without local telemetry: A generalized model using satellite data. Solar Energy, 172, 45-56.
- Solar photovoltaic generation forecasting methods: A review. Renewable and Sustainable Energy Reviews, 86, 1045-1061.
- Landsat and MODIS satellite image processing for solar irradiance estimation in the department of Narino-Colombia. Remote Sensing Applications: Society and Environment, 12, 100235.
- Automatic solar photovoltaic panel detection in satellite imagery. IEEE Journal of Selected Topics in Applied Earth Observations and Remote Sensing, 8(10), 4623-4633.
- Estimating residential building energy consumption using overhead imagery. Applied Energy, 280, 116018.
- Exploring Renewable Energy Resources Using Remote Sensing and GIS—A Review. Resources, 8(3), 149.
- Vegetation management of utility corridors using high-resolution hyperspectral imaging and LiDAR. Remote Sensing, 8(9), 756.
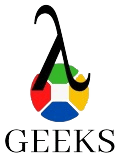
The lambdageeks.com Core SME Team is a group of experienced subject matter experts from diverse scientific and technical fields including Physics, Chemistry, Technology,Electronics & Electrical Engineering, Automotive, Mechanical Engineering. Our team collaborates to create high-quality, well-researched articles on a wide range of science and technology topics for the lambdageeks.com website.
All Our Senior SME are having more than 7 Years of experience in the respective fields . They are either Working Industry Professionals or assocaited With different Universities. Refer Our Authors Page to get to know About our Core SMEs.