Nuclear energy-based medical diagnostic tools have revolutionized the field of healthcare, providing healthcare professionals with advanced imaging techniques and accurate diagnostic capabilities. Designing these tools requires a multidisciplinary approach, combining expertise in nuclear energy, medical physics, and artificial intelligence (AI). This comprehensive guide will delve into the key considerations, best practices, and technical details necessary for designing effective nuclear energy-based medical diagnostic tools.
Nuclear Reactor Design for Medical Isotope Production
The design of the nuclear reactor is a crucial component in the development of nuclear energy-based medical diagnostic tools. The reactor’s core geometry, fuel type, and neutron moderator all play a significant role in the production of the desired medical isotopes, such as molybdenum-99 (Mo-99) and technetium-99m (Tc-99m).
Reactor Core Geometry
The reactor core’s geometry can be optimized to enhance the neutron economy and the production of medical isotopes. One common design is the TRIGA (Training, Research, Isotopes, General Atomics) reactor, which features a cylindrical core with a graphite reflector. The TRIGA reactor design allows for the efficient production of medical isotopes while maintaining a high level of safety.
The core geometry can be described using the following formula:
V = π * r^2 * h
Where:
– V
is the volume of the reactor core
– r
is the radius of the reactor core
– h
is the height of the reactor core
By adjusting the values of r
and h
, the reactor core volume can be optimized to maximize the production of medical isotopes.
Fuel Type and Neutron Moderator
The choice of fuel type and neutron moderator also plays a crucial role in the reactor’s performance. Typically, research reactors used for medical isotope production utilize low-enriched uranium (LEU) fuel, which is safer and more proliferation-resistant than highly-enriched uranium (HEU) fuel.
The neutron moderator, such as light water or graphite, is responsible for slowing down the fast neutrons produced in the reactor core, allowing for more efficient neutron capture and the production of medical isotopes. The choice of moderator material is based on factors such as neutron scattering and absorption cross-sections, as well as the desired neutron energy spectrum.
Neutron Economy Optimization
To optimize the production of medical isotopes, the neutron economy of the reactor must be carefully managed. This involves balancing the neutron flux, neutron capture cross-sections, and the overall neutron utilization within the reactor core. Numerical simulations using tools like MCNP (Monte Carlo N-Particle Transport Code) can be employed to model the neutron behavior and optimize the reactor design for medical isotope production.
Radiation Shielding for Safety
Proper radiation shielding is essential to protect patients, medical staff, and the general public from unnecessary radiation exposure when using nuclear energy-based medical diagnostic tools. The thickness and composition of the shielding material must be carefully calculated to attenuate the radiation to acceptable levels.
The required thickness (d) of a shielding material to reduce the radiation intensity (I) to a fraction (f) of the initial intensity (I0) can be calculated using the following formula:
d = (1/μ) * ln(I0/I)
Where:
– d
is the thickness of the shielding material
– μ
is the linear attenuation coefficient of the shielding material for the specific type of radiation
The linear attenuation coefficient (μ) depends on the energy of the radiation and the composition of the shielding material. Common shielding materials used in nuclear medicine include lead, concrete, and high-density polyethylene.
To ensure the effectiveness of the radiation shielding, it is essential to conduct detailed calculations and simulations to determine the optimal thickness and composition of the shielding materials. This can be done using software tools like MCNP or SHIELD-HPC, which can model the radiation transport and shielding performance.
AI-Powered Image Analysis for Diagnostic Accuracy
Artificial intelligence (AI) algorithms, particularly deep learning models, have revolutionized the field of medical image analysis. These algorithms can be trained to detect patterns and anomalies in medical images, providing valuable information to healthcare professionals for accurate diagnosis and treatment planning.
Deep Learning for Medical Image Analysis
Deep learning models, such as convolutional neural networks (CNNs), have demonstrated exceptional performance in tasks like tumor detection, organ segmentation, and disease classification in nuclear medicine imaging. These models can be trained on large datasets of medical images to learn the relevant features and patterns that are indicative of various medical conditions.
The performance of these AI algorithms can be evaluated using metrics such as accuracy, precision, recall, and F1-score. The RELAINCE (Radiology and Artificial Intelligence in Nuclear Medicine and Imaging) guidelines provide a comprehensive framework for evaluating AI algorithms in nuclear medicine, ensuring their reliability and clinical utility.
Integrating AI with Nuclear Medicine Workflows
To effectively integrate AI-powered image analysis into nuclear energy-based medical diagnostic tools, it is crucial to consider the workflow and data management aspects. This includes:
- Data Collection and Curation: Establishing a robust data collection and curation process to ensure the availability of high-quality, diverse medical images for training and validating the AI models.
- Model Training and Deployment: Developing and training the AI models using state-of-the-art deep learning techniques, and deploying them seamlessly within the nuclear medicine diagnostic workflow.
- Continuous Improvement: Implementing a feedback loop to continuously monitor the performance of the AI models and make necessary adjustments to improve their accuracy and reliability over time.
By integrating AI-powered image analysis with nuclear energy-based medical diagnostic tools, healthcare professionals can leverage the power of advanced imaging techniques and automated decision support to enhance diagnostic accuracy and patient outcomes.
Data Collection and Analysis for Quality Improvement
Quality improvement activities in nuclear medicine laboratories should emphasize accuracy, efficiency, patient and staff safety, and the patient’s experience during care. Collecting and analyzing data is crucial for identifying areas for improvement and monitoring the effectiveness of interventions.
Stratified Random Sampling
To ensure that the data collected is representative of the entire population, techniques like stratified random sampling can be employed. This involves dividing the population into homogeneous subgroups (strata) based on relevant characteristics, such as patient demographics or imaging modalities, and then randomly selecting samples from each stratum.
The formula for calculating the sample size (n) for stratified random sampling is:
n = Σ (Nh * √(ph * (1 - ph)) / E)^2
Where:
– Nh
is the size of the hth stratum
– ph
is the proportion of the characteristic of interest in the hth stratum
– E
is the desired margin of error
By using stratified random sampling, the data collected can better represent the diversity of the patient population and provide more accurate insights for quality improvement initiatives.
Data Analysis Techniques
Various data analysis techniques can be employed to identify areas for improvement and monitor the effectiveness of interventions in nuclear medicine laboratories. These include:
- Descriptive Statistics: Calculating measures of central tendency (mean, median, mode) and dispersion (standard deviation, range) to understand the distribution of the data.
- Inferential Statistics: Conducting hypothesis tests and regression analysis to identify statistically significant relationships between variables and assess the impact of interventions.
- Process Control Charts: Utilizing control charts, such as X-bar and R charts, to monitor the stability and variability of key performance indicators over time.
By leveraging these data collection and analysis techniques, nuclear medicine laboratories can make data-driven decisions, implement targeted improvements, and enhance the overall quality and safety of their diagnostic services.
Conclusion
Designing nuclear energy-based medical diagnostic tools requires a comprehensive understanding of nuclear reactor design, radiation shielding, AI-powered image analysis, and data-driven quality improvement. By following the best practices and technical details outlined in this guide, healthcare professionals and researchers can develop innovative and effective nuclear energy-based diagnostic tools that improve patient outcomes and advance the field of nuclear medicine.
References
- Jha, A. K., et al. (2023). Nuclear Medicine and Artificial Intelligence: Best Practices for Evaluation (the RELAINCE Guidelines). Journal of Nuclear Medicine, 64(1), 11-18.
- Kollias, S., et al. (2022). Machine learning for analysis of real nuclear plant data in the frequency domain. Annals of Nuclear Energy, 165, 108718.
- Nuclear Regulatory Commission. (2014). Introduction to Advanced Diagnostics and Prognostics for NRC Staff. Technical Report.
- SNM Journals. (2012). A Practical Guide to Quality Improvement in Nuclear Medicine. Journal of Nuclear Medicine Technology, 40(1), 1-12.
- Duderstadt, J. J., & Hamilton, L. J. (1976). Nuclear Reactor Analysis. Wiley.
- Knoll, G. F. (2010). Radiation Detection and Measurement. Wiley.
- Seibert, J. A., & Boone, J. M. (2005). X-ray imaging physics for nuclear medicine technologists. Part 2: X-ray interactions and image formation. Journal of Nuclear Medicine Technology, 33(1), 3-18.
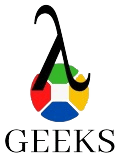
The lambdageeks.com Core SME Team is a group of experienced subject matter experts from diverse scientific and technical fields including Physics, Chemistry, Technology,Electronics & Electrical Engineering, Automotive, Mechanical Engineering. Our team collaborates to create high-quality, well-researched articles on a wide range of science and technology topics for the lambdageeks.com website.
All Our Senior SME are having more than 7 Years of experience in the respective fields . They are either Working Industry Professionals or assocaited With different Universities. Refer Our Authors Page to get to know About our Core SMEs.