The axioms of probability are fundamental principles that form the basis of probability theory. These axioms provide a set of rules for assigning probabilities to events. By following these rules, we can make accurate predictions and analyze uncertain outcomes. The three axioms of probability are:
- Non-Negativity: The probability of an event is always greater than or equal to zero. It cannot be negative.
- Additivity: For mutually exclusive events, the probability of their union is equal to the sum of their individual probabilities.
- Normalization: The probability of the entire sample space is equal to one. In other words, the sum of the probabilities of all possible outcomes is equal to one.
Key Takeaways
Axiom | Description |
---|---|
Non-Negativity | Probability of an event is always greater than or equal to zero. |
Additivity | Probability of mutually exclusive events is the sum of their individual probabilities. |
Normalization | The sum of probabilities of all possible outcomes is equal to one. |
Understanding the Concept of Probability

Probability is a fundamental concept in the field of mathematics and statistics. It allows us to quantify the likelihood of events occurring and make informed decisions based on that information. Whether you realize it or not, probability plays a significant role in our everyday lives. From predicting the weather to making financial investments, understanding probability is essential for making sense of the uncertain world around us.
Definition of Probability
At its core, probability is a measure of the likelihood of an event occurring. It is represented as a number between 0 and 1, where 0 indicates impossibility and 1 represents certainty. The concept of probability is based on the idea of randomness, where outcomes are uncertain and can be influenced by various factors.
To formalize the study of probability, mathematicians have developed Probability Theory, which is built upon Kolmogorov’s axioms. These axioms provide a mathematical framework for defining probability and studying its properties. Probability theory allows us to analyze and understand the behavior of random events and make predictions based on statistical principles.
Importance of Probability in Real Life
Probability is not just a theoretical concept; it has practical applications in various aspects of our lives. Let’s explore some areas where probability plays a crucial role:
Risk Assessment: Probability is used to assess risks in fields such as insurance, finance, and healthcare. By analyzing historical data and using mathematical statistics, probabilities can be assigned to different outcomes, helping individuals and organizations make informed decisions.
Data Analysis: Probability is an essential tool in data analysis. It helps us understand the likelihood of certain events occurring and enables us to make predictions based on available data. Concepts like conditional probability, probability distribution, and Bayes’ theorem are used to analyze data and draw meaningful insights.
Decision Making: Probability allows us to make rational decisions in the face of uncertainty. By considering the probabilities of different outcomes, we can evaluate the potential risks and rewards associated with each decision. This is particularly useful in fields like business, where decisions can have significant financial implications.
Predictive Modeling: Probability is used extensively in predictive modeling, where the goal is to forecast future events based on historical data. Techniques like the binomial distribution, law of large numbers, and central limit theorem are employed to build models that can make accurate predictions.
The Three Axioms of Probability
Axiom 1: The Probability of an Event
In probability theory, we often deal with the likelihood of certain events occurring. These events can range from simple outcomes, such as flipping a coin and getting heads, to more complex scenarios, like predicting the outcome of a sports game. To make sense of these probabilities, mathematician Andrey Kolmogorov introduced three fundamental principles, known as Kolmogorov’s axioms.
The first axiom, known as the Probability of an Event, states that the probability of any event occurring is a number between 0 and 1, inclusive. A probability of 0 means that the event is impossible, while a probability of 1 means that the event is certain to happen. For example, if we toss a fair six-sided die, the probability of rolling a 1 is 1/6, as there is only one favorable outcome out of six possible outcomes.
Axiom 2: The Probability of the Sample Space
Moving on to the second axiom, we have the Probability of the Sample Space. The sample space refers to the set of all possible outcomes of an experiment or event. It encompasses every possible result that could occur. According to this axiom, the probability of the sample space is equal to 1. In other words, the sum of the probabilities of all possible outcomes within the sample space must add up to 1. This axiom ensures that the total probability is accounted for and provides a foundation for further calculations.
To better understand this concept, let’s consider a simple example. Suppose we have a dataset of employees and we want to calculate the probability of an employee being salaried or hourly. The sample space in this case would consist of two possible outcomes: salaried or hourly. The probability of the sample space, which includes both outcomes, would be equal to 1.
Axiom 3: The Probability of Any Number of Mutually Exclusive Events
The third axiom deals with the probability of any number of mutually exclusive events. Mutually exclusive events are events that cannot occur simultaneously. For example, if we roll a six-sided die, the events of rolling a 1 and rolling a 2 are mutually exclusive because they cannot happen at the same time.
According to this axiom, the probability of the union of mutually exclusive events is equal to the sum of their individual probabilities. In other words, if we have two or more mutually exclusive events, we can calculate the probability of their occurrence by adding up their individual probabilities. This axiom allows us to analyze and calculate probabilities for more complex scenarios involving multiple events.
Probability theory is a fundamental concept in mathematics and plays a crucial role in various fields, including data analysis, mathematical statistics, and stochastic processes. Understanding the three axioms of probability is essential for grasping the concept of probability and applying it effectively in real-world scenarios.
Now that we have explored the three axioms of probability, we can delve deeper into other related topics, such as conditional probability, probability distributions, randomness, statistical independence, Bayes’ theorem, random variables, cumulative distribution functions, probability density functions, expected values, and more. These concepts build upon the foundation laid by the axioms and allow for more advanced calculations and analysis.
Importance of Axioms of Probability
Role of Axioms in Probability Theory
In the field of probability theory, axioms play a crucial role in establishing a solid foundation for the study of randomness and uncertainty. These axioms, specifically Kolmogorov’s axioms, provide a set of fundamental principles that guide the mathematical treatment of probabilities. By defining the rules and properties of probability, axioms ensure that the calculations and interpretations of probabilities are consistent and reliable.
To understand the significance of axioms in probability theory, let’s delve into the reasons why we need them.
Why We Need Axioms of Probability
1. Establishing a Framework for Analysis
Axioms of probability provide a framework for analyzing uncertain events and their associated probabilities. They define the basic concepts and rules that allow us to quantify the likelihood of different outcomes. By providing a common language and set of principles, axioms enable researchers and statisticians to communicate and collaborate effectively in the field of probability theory.
2. Ensuring Consistency and Coherence
Axioms ensure that the calculations and interpretations of probabilities are consistent and coherent. They define the sample space, which represents all possible outcomes of an experiment, and the event space, which consists of subsets of the sample space representing specific events. By specifying the rules for assigning probabilities to events, axioms ensure that the probabilities assigned are meaningful and adhere to logical principles.
3. Facilitating Statistical Analysis
Axioms of probability are essential for conducting statistical analysis. They form the basis for mathematical statistics, which involves the use of probability theory to analyze and interpret data. Concepts such as conditional probability, probability distribution, randomness, and statistical independence rely on the axioms of probability. These concepts are crucial for understanding and modeling real-world phenomena, making predictions, and making informed decisions based on data.
4. Enabling Bayesian Inference
Detailed Explanation of Axioms of Probability
Probability theory is a fundamental concept in mathematical statistics and plays a crucial role in understanding randomness and uncertainty. Kolmogorov’s axioms provide a solid foundation for probability theory, ensuring that the rules and principles of probability are well-defined and consistent. In this detailed explanation, we will explore the three axioms of probability and their significance in the field of statistics.
Understanding Axiom 1 with Examples
Axiom 1, also known as the “Non-Negativity Axiom,” states that the probability of any event is a non-negative number. In other words, the probability of an event cannot be negative. It is always equal to or greater than zero.
To illustrate this axiom, let’s consider a simple example. Suppose we have a dataset of employees in a company, and we want to analyze the probability of an employee being salaried. The event “being salaried” can be denoted as E. According to Axiom 1, the probability of event E, denoted as P(E), must be greater than or equal to zero.
Understanding Axiom 2 with Examples
Axiom 2, also known as the “Normalization Axiom,” states that the probability of the entire sample space is equal to one. The sample space represents all possible outcomes of an experiment or event. It encompasses every possible outcome that could occur.
To better understand this axiom, let’s consider a scenario where we are analyzing customer churn in a telecommunications company. The sample space consists of two possible outcomes: “churn” and “not churn.” Denoting “churn” as A and “not churn” as B, the probability of the sample space (A ∪ B) is equal to one, as per Axiom 2.
Understanding Axiom 3 with Examples
Axiom 3, also known as the “Additivity Axiom” or “Probability Sum Rule,” states that the probability of the union of two or more mutually exclusive events is equal to the sum of their individual probabilities. Mutually exclusive events are events that cannot occur simultaneously.
Let’s consider a practical example to illustrate this axiom. Suppose we are analyzing the probability of a customer having either a one-year contract or a two-year contract with a telecommunications company. Denoting the event “one-year contract” as C and the event “two-year contract” as D, the probability of either event occurring (C ∪ D) is equal to the sum of their individual probabilities, as stated by Axiom 3.
Proving the Axioms of Probability
Probability theory is a fundamental concept in mathematical statistics and plays a crucial role in various fields such as data analysis and stochastic processes. To establish a solid foundation for probability theory, we rely on Kolmogorov’s axioms. These axioms provide a set of rules that define the behavior of probabilities and enable us to make meaningful calculations and predictions.
How to Prove Axiom 1
Axiom 1 states that the probability of any event is a non-negative number. To prove this axiom, we need to show that the probability of an event cannot be negative. Let’s consider a probability space, which consists of a sample space and an event space. The sample space represents all possible outcomes of an experiment, while the event space contains subsets of the sample space that we are interested in.
To demonstrate that probabilities are non-negative, we can use a simple example. Suppose we have a dataset of employees, and we want to calculate the probability of an employee being salaried. Since the concept of probability is based on the frequency of events, we can count the number of salaried employees and divide it by the total number of employees. The resulting probability will always be a non-negative value, as it represents the proportion of salaried employees in the dataset.
How to Prove Axiom 2
Axiom 2 deals with the probability of the entire sample space, which is always equal to 1. To prove this axiom, we need to show that the sum of probabilities of all possible outcomes is equal to 1. This axiom ensures that the total probability is distributed among all possible outcomes.
To illustrate this, let’s consider a churn analysis scenario. We have a dataset of customers, and we want to calculate the probability of a customer churning. In this case, the sample space consists of two outcomes: churn and non-churn. The probability of churn plus the probability of non-churn will always equal 1, as these two outcomes encompass all possibilities.
How to Prove Axiom 3
Axiom 3 deals with the probability of the intersection of two or more events. It states that the probability of the intersection of two events is equal to the product of their individual probabilities. To prove this axiom, we need to show that the probability of the intersection of two events is calculated by multiplying their probabilities.
To understand this concept, let’s consider a scenario where we want to calculate the probability of both event A and event B occurring. We can calculate the probability of event A and multiply it by the probability of event B, resulting in the probability of their intersection. This axiom is essential in determining the joint probability of multiple events and is frequently used in statistical analysis.
By proving these three axioms, we establish the foundation of probability theory. These axioms allow us to define the behavior of probabilities, calculate conditional probabilities, explore probability distributions, and apply concepts such as randomness and statistical independence. They also form the basis for important theorems like Bayes’ theorem and concepts like random variables, cumulative distribution functions, probability density functions, expected values, and more.
Axioms of Probability in Different Fields
Axioms of Probability in Statistics
When it comes to probability theory, there are certain fundamental principles that serve as the building blocks for understanding and analyzing random events. These principles, known as the axioms of probability, provide a solid foundation for statistical analysis and decision-making. Let’s explore the axioms of probability in different fields, starting with statistics.
In statistics, probability theory is used to quantify uncertainty and make informed predictions based on data. The axioms of probability in statistics are as follows:
Sample Space: The sample space refers to the set of all possible outcomes of an experiment. It is denoted by the symbol Ω and represents the universe of events under consideration.
Event Space: The event space consists of subsets of the sample space, representing specific outcomes or combinations of outcomes. An event is a collection of one or more outcomes. It is denoted by the symbol E and can range from a single outcome to the entire sample space.
Probability Function: The probability function assigns a numerical value to each event in the event space, representing the likelihood of that event occurring. It satisfies the following conditions:
- The probability of any event is a non-negative number.
- The probability of the entire sample space is equal to 1.
The probability of the union of mutually exclusive events is equal to the sum of their individual probabilities.
Conditional Probability: Conditional probability measures the likelihood of an event occurring given that another event has already occurred. It is denoted by P(A|B), where A and B are events. The conditional probability satisfies the following formula: P(A|B) = P(A ∩ B) / P(B).
Independence: Two events A and B are considered independent if the occurrence of one event does not affect the probability of the other event. Mathematically, this can be expressed as P(A ∩ B) = P(A) * P(B).
Axioms of Probability in Artificial Intelligence
In the field of artificial intelligence, probability theory plays a crucial role in modeling uncertainty and making informed decisions. The axioms of probability in artificial intelligence build upon the foundations laid by Kolmogorov’s axioms and extend them to suit the specific needs of AI applications. Let’s delve into the axioms of probability in artificial intelligence:
Probability Distribution: A probability distribution describes the likelihood of each possible outcome in a random variable. It provides a complete summary of the probabilities associated with each value or range of values that the random variable can take.
Random Variables: Random variables are variables whose values are determined by the outcome of a random event. They can be discrete or continuous, depending on whether they take on a countable or uncountable set of values.
Cumulative Distribution Function (CDF): The cumulative distribution function gives the probability that a random variable takes on a value less than or equal to a given value. It provides a comprehensive view of the probabilities associated with different values of the random variable.
Probability Density Function (PDF): The probability density function describes the probability of a continuous random variable taking on a specific value. It is used to calculate the probability of the random variable falling within a certain range.
Expected Value: The expected value of a random variable is the average value it would take over an infinite number of repetitions of the random experiment. It is a measure of the central tendency of the random variable.
Bayes’ Theorem: Bayes’ theorem is a fundamental concept in probability theory that allows us to update our beliefs about an event based on new evidence. It provides a framework for incorporating prior knowledge and updating it with observed data.
These axioms of probability in different fields form the backbone of statistical analysis, data modeling, and decision-making in various domains. Understanding these principles is essential for making sense of the uncertain and complex nature of real-world phenomena. Whether you are analyzing data, building AI models, or making predictions, probability theory provides the tools to navigate the realm of uncertainty and make informed choices.
What is the relationship between the axioms of probability and the fundamental axioms of mathematics?
The axioms of probability, as discussed in https://techiescience.com/axioms-of-probability/, define the foundational principles that govern the field of probability theory. On the other hand, the concept of axioms in mathematics is a fundamental aspect that establishes the basis of mathematical reasoning and proof. By exploring the fundamental axioms of mathematics, we can uncover the connections and dependencies they share with the axioms of probability. These intersections allow us to understand how mathematical principles underpin the rules and framework of probability theory, providing a solid foundation for probabilistic reasoning and analysis of uncertain events.
Frequently Asked Questions
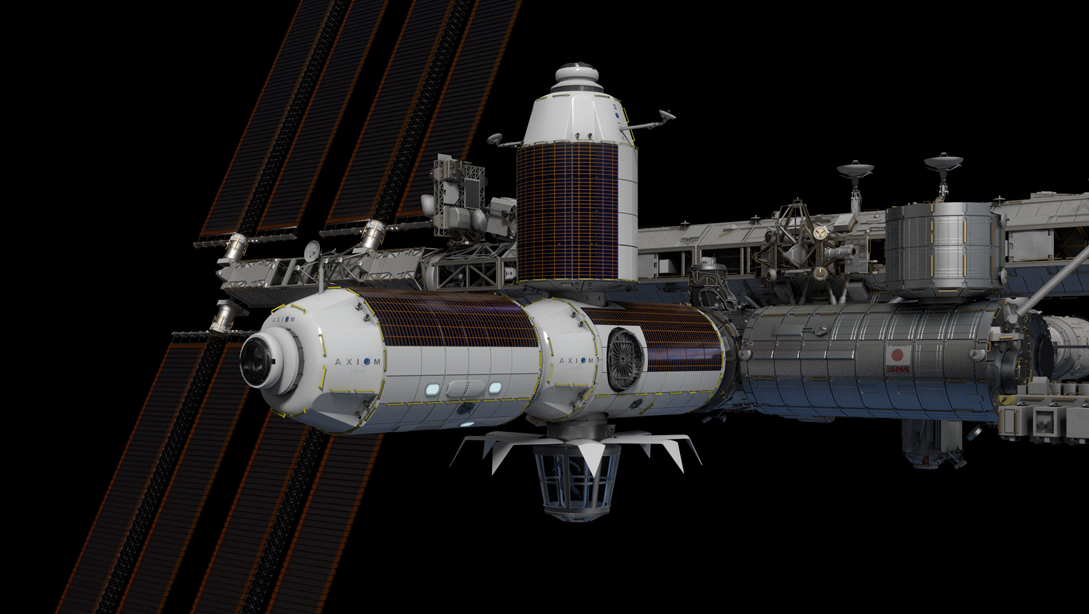
Q1: What are the 3 Axioms of Probability?
Answer: The three axioms of probability are:
1. Non-negativity: The probability of any event is always non-negative.
2. Normalization: The probability of the entire sample space is equal to 1.
3. Additivity: If two events are mutually exclusive, the probability of the occurrence of at least one event is the sum of their individual probabilities.
Q2: Why is Axiom of Probability Important?
Answer: The axioms of probability provide a solid foundation for the mathematical theory of probability. They help us to define and measure the likelihood of events in a consistent and logical manner, which is essential in many fields, including statistics, computer science, and artificial intelligence.
Q3: What Does Axioms of Probability Mean in Statistics?
Answer: In statistics, the axioms of probability provide a framework to calculate the probability of events. They are the basic assumptions on which the entire theory of probability is built. They help us to predict the likelihood of an event occurring based on the outcomes of a random experiment.
Q4: What is Axioms of Probability in Artificial Intelligence?
Answer: In the field of artificial intelligence, the axioms of probability are used to model uncertainty. They play a crucial role in probabilistic reasoning, which is a key aspect of many AI algorithms, including Bayesian networks and Markov decision processes.
Q5: How to Prove Axioms of Probability?
Answer: The axioms of probability are generally accepted as self-evident and do not require proof. However, their implications and the derived laws of probability, such as Bayes’ theorem and the law of total probability, can be proven using these axioms as a foundation.
Q6: Why Do We Need Axioms of Probability?
Answer: We need the axioms of probability to provide a mathematical framework for dealing with uncertainty. They enable us to calculate and predict the likelihood of events in a consistent and logical manner, which is crucial in many fields, including science, engineering, economics, and social sciences.
Q7: List the Axioms of Probability.
Answer: The three axioms of probability are:
1. Non-negativity: The probability of an event is always non-negative.
2. Normalization: The probability of the entire sample space is equal to 1.
3. Additivity: The probability of the union of two mutually exclusive events is the sum of their individual probabilities.
Q8: How to Show Axioms of Probability?
Answer: The axioms of probability can be shown or demonstrated through a probability space, which consists of a sample space, an event space, and a probability measure that assigns probabilities to events in accordance with the axioms.
Q9: What is the Probability of an Event?
Answer: The probability of an event is a measure of the likelihood that the event will occur. It is calculated as the ratio of the number of favorable outcomes to the total number of possible outcomes in the sample space.
Q10: What is the Axiom of Probability 1?
Answer: The first axiom of probability, also known as the axiom of non-negativity, states that the probability of any event is a non-negative real number. This means that probabilities are always between 0 and 1, inclusive.
Also Read:
- Autorefractor
- Mesosphere 2
- Irrational number
- Dioptric power
- Hydrosphere
- Axioms
- Prism 2
- Quotient rule
- Element 115
- Exergonic vs endergonic

The TechieScience Core SME Team is a group of experienced subject matter experts from diverse scientific and technical fields including Physics, Chemistry, Technology,Electronics & Electrical Engineering, Automotive, Mechanical Engineering. Our team collaborates to create high-quality, well-researched articles on a wide range of science and technology topics for the TechieScience.com website.
All Our Senior SME are having more than 7 Years of experience in the respective fields . They are either Working Industry Professionals or assocaited With different Universities. Refer Our Authors Page to get to know About our Core SMEs.